How Leaders Should Think About Artificial Intelligence and What They Should Do
Published on Nov 28, 2022
16 min read
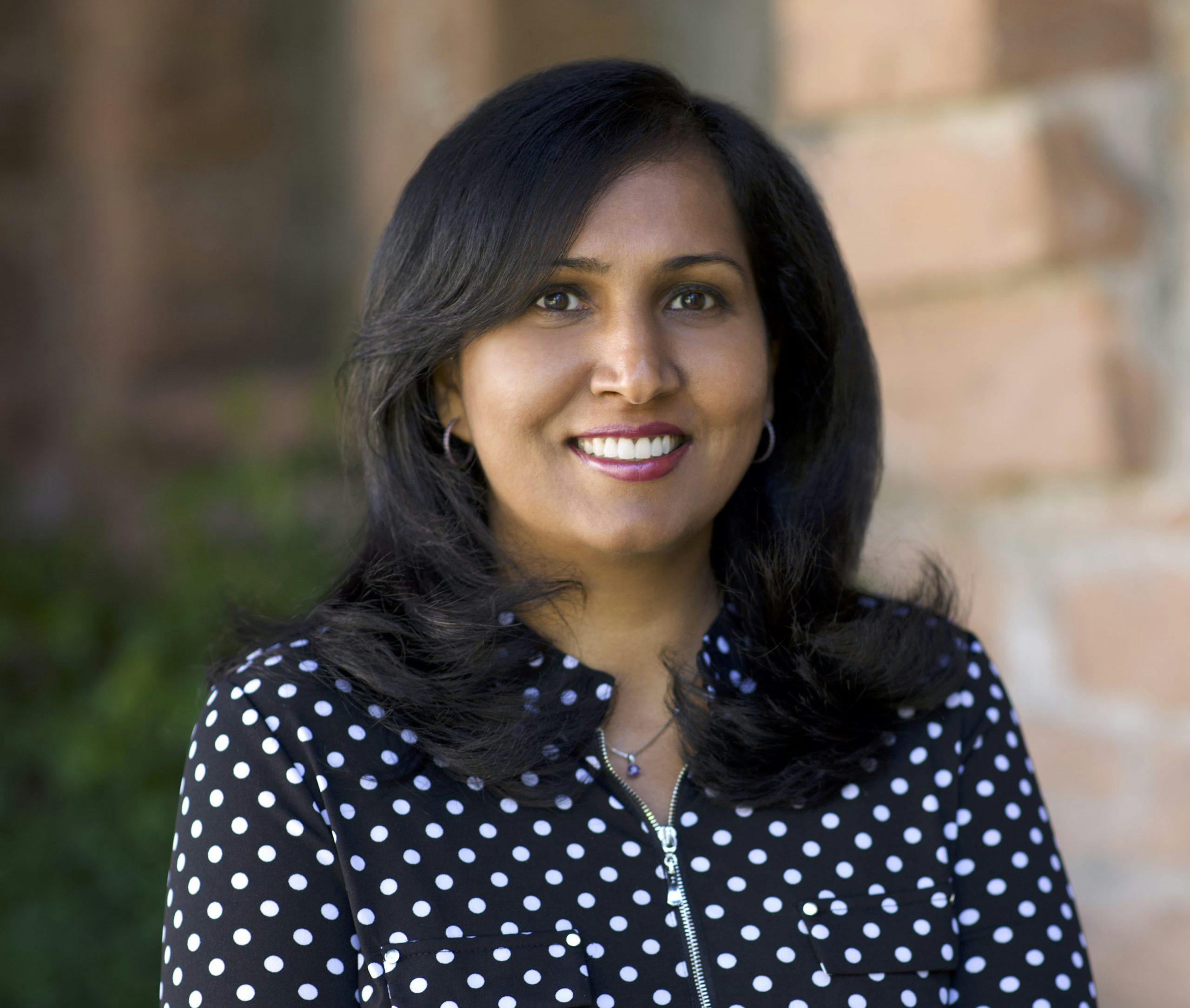
Many business leaders struggle to wrap their heads around artificial intelligence and what it could mean for their companies. In this video, Kavita Ganesan, author of The Business Case for AI, will explore how leaders should think about AI and what they should do to ensure that their companies are prepared for the future. We’ll also bust some of the most common AI myths and show how businesses can benefit from AI. So if you’re a leader who wants to stay ahead of the curve, this video is a must-watch!
[https://youtu.be/JMriGMRq7kU](https://youtu.be/JMriGMRq7kU "Play video "Artificial Intelligence: What Leaders Need to Know"")
Video can’t be loaded because JavaScript is disabled: Artificial Intelligence: What Leaders Need to Know (https://youtu.be/JMriGMRq7kU)
**Have suggestions for future podcast guests or other feedback? Let me know here.
**Subscribe to the ManagersClub show: Apple Podcasts | Google Podcasts | Spotify | YouTube
CHAPTERS
- 0:58 Introduction
- 3:59 What’s different now, in 2022, that people should pay attention to AI?
- 5:00 What are the myths and misunderstandings about AI?
- 7:57 How should engineering leaders think about adopting AI and integrating it in?
- 9:38 How should you measure the success of an AI project?
- 11:50 How can a company be AI-ready?
- 14:12 How should leaders spot AI opportunities and capitalize on them?
- 16:22 The dangerous pitfalls of AI for AI’s sake.
- 18:48 What resources would you recommend to leaders to become more knowledgeable in this area?
- 20:04 What will be the impact of AI on software engineering?
- 21:46 Where can people reach out to you afterward?
RESOURCES
- ⭐️ https://www.linkedin.com/in/kavita-ganesan
- ⭐️ The Business Case for AI: A Leader’s Guide to AI Strategies, Best Practices & Real-World Applications: https://amzn.to/3GLJmjM
- ⭐️ https://kavita-ganesan.com/
[powerpress]
Transcript
Kavita: [00:00:00] there’s a lot of misunderstanding on what AI really is, uh, like on the leadership side versus the implementation side.
how do you find AI opportunities? How do you measure the success of AI? Should you build or should you buy? So all these topics are very relevant to leaders and I think there’s a lot of misunderstanding
so the way I see AI success is based on three core pillars…
Vidal: Just a very quick thing before we get started. If you’re new here, my name is Vidal and you’re listening to the Managers Club [00:00:30] podcast, where I interview remarkable managers and inspiring leaders. We discuss how they got to where they are to gain insights to advance your career. You’re about to hear a conversation between me and Kavita Ganesan, author of the Business Case for AI.
We discuss how business leaders should think about artificial intelligence and dispel some common myths about ai. If you’re a leader who wants to be prepared to take advantage of AI, this video is for you.
Good afternoon. Today I [00:01:00] have with me Kavita Ganesan, this is a really special treat. We’re gonna talk about AI today. So, uh, Kavita, welcome to Managers Club.
Kavita: Yeah, thank you for having me. Vidal.
Vidal: Yeah. Um, Kavita, this is such a timely topic now for all engineering leaders and I was looking at your background.
You have a very strong background in AI. You have a PhD in it. Tell us a little bit about your background and, uh, why did you decide to write this book?
Kavita: Yeah, so I’ve been, um, in AI for a long time now like since [00:01:30] 2005. I really got my start, um, when I joined, uh, USC for my master’s program. And I was introduced to this whole world of ai, uh, because they have a really strong, uh, AI department.
And, um, so I learned about algorithm development and there was a lot of creativity and problem solving going on, and that really, uh, resonated with me because I really like problem solving. And I also like the [00:02:00] creative thinking aspect, which goes along with ai. So you have to be creative in coming up with algorithms.
So that’s how I got hoped to, uh, AI and then I went on to doing my PhD in the field, um, specializing in natural language processing and machine learning, but more on the applied side of things. Um, and then when I graduated, um, data science was really taking off the rest around 2013. So I [00:02:30] went on to solving a bunch of industry problems, like on a hands-on capacity.
And then, um, more and more people were contacting me, like just out of the blue to help them implement AI in their company. So that’s how I got more into consulting. And I was able to see a lot of the problems in the field.
Like there’s a lot of misunderstanding on what AI really is, uh, like on the leadership side versus the implementation side. So I wanted a book to [00:03:00] be a bridge. So right in between let leaders understand AI at a high level without going into the technical details. So that’s how my book got its start and actually use it now with, uh, my clients.
And the book doesn’t assume that, you know, ai it start off, uh, starts off with a very foundation of what is. And then it works its way up to like, um, how do you find AI opportunities? How do you measure the success of ai? Should you build [00:03:30] or should you buy? So all these topics are very relevant to leaders and I think there’s a lot of misunderstanding, um, right there.
So I try to address a lot of that in my book.
Vidal: I think it’s great. Yes. I love that the book is for leaders, so things can be really, really relevant for people mm-hmm. On, uh, managers club, and your book also super practical. Mm-hmm. Like it has a lot of very practical actionable advice. So let me just start with the easy question here.
So, you know, we’ve been here about AI for a long time. Okay? It’s not a new [00:04:00] thing. Mm-hmm. But, um, what’s different now in 2022, um, that, that people should pay attention? Like, for example, I’ve been playing with a G P T 3 and things like that, so talk a little bit about that.
Kavita: What’s different now is the layers and layers of abstraction that has gone over the, um, hardcore a.
So right now you can use GPT-3 to make sentiment predictions with like three lines of code. So there are lots of tools, more and more tools available [00:04:30] that you can easily plug into different systems. So this was not the case in 2005. You actually had to write a lot of things from scratch. Even the Python tools that you have right now, you didn’t have back then in 2005, that all came more.
So tools are more available, but I, the problems that I see now are using those tools for the right use cases and in the right way that you actually get value from them. [00:05:00]
Vidal: Okay. Um, You talk about the myths around AI, the myths, the hype, the misunderstanding, and also what’s realistic. So, uh, for leaders, could you discuss a little bit like what are the, the myths and misunderstandings about AI today?
Kavita: Yeah. A common myth I see on the engineering leadership side is that AI systems should work, uh, 99% of the time accurately out of the. That’s really not true because [00:05:30] AI systems are trained on specific type of data, and when you try to use them on your data, it may not look the same. The accuracy might not be the same, so there’s a high chance that the accuracy drops to maybe from 99% to 60%, and you need to be prepared for these kind of errors that you.
So even self-driving cars make errors all the time. They may not recognize objects and they might just, you have heard of accidents [00:06:00] from self-driving cars because they didn’t see the object. So there are many problems like that, uh, where people think that AI systems should, uh, work out of the box. But it really, um, it’s all about testing, evaluating, setting your expectations, right, and, uh, um, just making sure it works in your particular, Yeah, that’s one.
Yeah,
Vidal: go ahead. There. There are more myths?
Kavita: Yes, there’s more myths. Yeah. Um, [00:06:30] another myth I commonly see on the engineering side is that sophistication is superior. So of course you have tools like GPT-3 now available that you can just plug in so people want to use that right um, for every single problem but that might not be the best tool to use because the newer tools are harder to productionize. Uh, they might be a lot more expensive than the simpler machine learning tools that like maybe logistic regression. [00:07:00] So that might be easier to use for that problem versus using GPT-3, which is expensive, harder to productionize, um, and may not really fit that particular use.
Vidal: Yeah. I think you even mentioned in the book, right, some cases are some attorneys like wanted to use ai, but you’re like, you don’t even need ai, you just need a software engineer to like write some automation.
Kavita: Correct. Yeah. So it was basically trying to autopopulate a pattern based on different [00:07:30] criteria.
So it was basically an if else problem versus an AI problem. So, but because it dealt with reading text from a data, So they categorized this as an NLP problem, but it wasn’t an NLP problem. It was a form filling problem based on your criteria. And then I tried to ask the I to not use ai, just use, uh, just get a software engineer to help you build out the tool.
Vidal: How should engineering leaders think about [00:08:00] adopting AI and integrating it in?
Kavita: Um, So it depends on the problem. So for some problems you may have to build the solution from scratch. Uh, so you’ll have to build a custom model, but for a lot of problems, the models are already out there. So, for example, translating from one language to another.
A lot of cloud tools available that you can integrate, [00:08:30] um, into your. So you want to first ensure that you, whether you really wanna develop from scratch or are tools already out there. And once you know which way you’re going, let’s say you’re trying to use an off the shelf solution, now you have to make sure that it actually works on your data.
So you have to, uh, test multiple solutions as opposed to just going with the cheapest or the most popular. So testing on your [00:09:00] data is very, very important. And then also establishing your metrics. So for your models, you may have certain metrics, but you want to also consider your business metrics. So what is the purpose of you using a particular model?
So are you trying to reduce the time to solve the specific tasks? Are you trying to improve response times? So understanding those metrics will allow you to see how this AI solution is actually working and helping the [00:09:30] business. So don’t just look at engineering problem, also look at the big picture. How is it gonna impact the business?
Vidal: Kavita, how do you measure the success of an AI project? You know, you’re an engineering leader and your team has an AI initiative or something.
How do you measure the success of it?
Kavita: So the way I see AI success is based on three core pillars. The first is model success. So the model has to do what it [00:10:00] promises to do. Um, so the accuracy of the model has to be, uh, acceptable both in development as well as in production.
Uh, but model is a means to an end, so it has to impact the business in some way. So that’s the whole point of developing the. So then for, you’ll also have to look at business success. So what are the metrics that are gonna be impacted by using the model? So you need [00:10:30] to be able to track those things. Um, so let’s say you’re trying to reduce the task to perform something.
So the time to task competition, what you should be tracking for on the business side of things. And once that’s, uh, that’s going well, then you also want to get. From the users. So that’s where user success really comes in, because if the users are not happy with the solution, there’s going to be user adoption risks.
Um, so for [00:11:00] example, if the user has to take 10 additional steps in order to consume your AI output, that’s introducing inefficiencies in their workflow. So it’s, uh, unlikely that they’ll use your solution five to 10 years from. So you want to also get user feedback. And user feedback can also reveal things that the models may have missed, right?
The model may be making mistakes on really simple things, so getting feedback from the users can iron out all those things. [00:11:30] And by evaluating these three success pillars, I think you are actually improving your chances of, um, the AI solution going into production and creating value for the business.
Vidal: That’s, that’s awesome.
I think it’s a great way to look at it. Thank you.
Um, what does it mean for a company to talk about this to be like AI ready?
Kavita: Mm-hmm. Yeah. So if you want to pilot one of, uh, [00:12:00] AI initiatives, you certainly. But if you want AI to be like, um, something that use a go-to tool for the long term, then you need to consider, uh, preparing your organization for ai and that can have different pillars.
So for example, you have the data readiness pillar. So this, for this, you have to consider questions like, are you aggressively collecting data within your throughout organization? And in some cases [00:12:30] big companies have data stores that are, that operate in silos. So you don’t get the whole picture of your data stores.
So are you warehousing data? Um, and some companies are still operating based off of paper processes. So for you to do any sort of analytics or ai, you need all that data in digital form. So have you digitized paper? So data is a big, uh, readiness element, uh, element that you should be considering early [00:13:00] on and on top of data.
There’s also cultural readiness, which I think is also pretty big because there’s a lot of fear around ai. Like all this fear brought out about these popular figures, like there will be AI takeover, we’ll have, uh, weaponization of ai. So there’s a lot of fear built into. Um, so we need to address those fear.
We need to be clear about how you’re going to use ai, for what purposes, what types of data will you use [00:13:30] or will you not use. Um, and so the ethical considerations are important and some of these can be addressed through education. Basically educating your employees and your customers about being transparent about how you use your, uh, ai and.
So other than this, uh, other elements like infrastructure, skills and budget. Uh, so some of these can be, um, [00:14:00] can be addressed as you are working on AI pilots, but I think the data readiness pillar and culture readiness, you need to be proactively working on, uh, for the long term. ,
Vidal: how should engineering leaders spot like AI opportunities and capitalize on them in a creative way?
Like there’s so many opportunities, like how should they, um, yeah, spot
Kavita: the right ones? So AI problems tend to solve like complex decision making problems [00:14:30] that require human like thinking, judgment, and the output can be very dynamic based on the input that’s. So, for example, uh, let’s say you’re a physician and you’re trying to predict the, a patient’s risk score of having lung cancer.
So you’re using, um, your years of training your subject matter expertise, uh, the patient’s, uh, chart information, uh, the patient’s health condition [00:15:00] essentially, and maybe the CT scans, um, generated for the patient. So using all of these data, To render a decision on a case by case basis for each and every patient, and one small abnormality in the CT scan may change your risk of prediction.
So this is a complex decision making problem, and you can teach a AI system to make similar predictions by showing it like historical examples of how [00:15:30] physicians have made, um, predictions on such similar health. So, so that these are the types of problems you want to look for. And AI problems are often like high volume and recurrent.
For it to make business sense, it has to be high volume and recurrent. Um, so another example is a recommendation, uh, system. So recommendation is not a one size fits all. It’s very personalized. And to do it manually, you’ll have to do this on a [00:16:00] case by case. So this is also in effect a complex decision making problem.
So these are the types of problems you want to find, like complex problems. Um, they are high volume, they’re recurring. And in my book, I actually have a framework to find this AI opportunities, like step by step framework, um, to do.
Vidal: Talk to me a little bit or about the pitfalls or like AI for AI’s sake.
So you had a great thing in the book where, you [00:16:30] know, people will make these sexy demos of stuff, you know, to draw attention, maybe to get themselves promoted and things like that. But at the end of the day, these, these demos don’t go anywhere. Mm-hmm. . So what should engineering leaders be careful of there?
Kavita: Yeah, so a lot of, uh, problems that come up, uh, within engineering teams is based on the data that’s available.
So you see some really nice large amounts of data, and then you start developing models. So maybe you have lots of [00:17:00] support tickets, and then you start developing sentiment classifiers over these support tickets. But really there is no real, uh, business use case for this model. So they develop and it’s production ready. And then they’re trying to shop like, who wants to use this model? Who wants, who needs it? So that really doesn’t work well because it doesn’t tie into any, uh, higher business goals or objectives. And it’s not solving like a [00:17:30] known high impact problem. And this happens a lot because systems need data, and that’s how engineers today come up with, uh, problems to solve based on what’s a.
So, but I think each the way should be the reverse. So we need leaders to say, um, what are some of the inefficiencies within different business functions? And they should be able to spot potential AI opportunities and then bring it to the data scientists. So let them assess if [00:18:00] these are AI solvable problems, and then look for the data to support the initiative.
And if the data’s not existent, that’s fine. You can start collecting right. So maybe you can get into the initiative six months down the road. So, but it should be more top down versus, uh, bottom up,
Vidal: really. Uh, I, I think that’s a great point you, you made, because one, the, these things are coming from the data scientists, machine learning engineers, maybe they’re, you know, [00:18:30] they’re not that useful.
So they’re coming from the leaders and the domain experts.
Kavita: So because they are sitting closer to the business challenges that’s happening. So they see the fire happening , so they need to bring the fire to the data scientist and say, Hey, is this a problem you can solve with ai?
Vidal: Awesome. Um, you know, besides your book, uh, which is an excellent book, what other resources would you recommend mentioning leaders if they want to, you know, become more knowledgeable in this area?[00:19:00]
Kavita: So, um, it depends on what type of engineering leader you are. So if you’re more on the high level site, I would recommend reading. Two to three different business books just to get different perspectives. Uh, but if you’re more hands on, then I would recommend reading, uh, books that are related to the specific tools that you would be using, uh, for your engineering problems.
And on top of that, uh, what I do is I set, uh, alerts because AI tools are changing [00:19:30] every day. So I set alerts for like AI tools, AI startups, which can give you an idea of where innovation is really happen. AI use cases. So I set different AI related alerts and that gives me the latest news. So that’s how I learn about how G P T three is progressing, what’s the latest out there.
So that’s a good way to keep up with the trend because AI changes every single day.
Vidal: I wanted to ask you another question. this is very close to my heart. Um, you know, [00:20:00] a lot of people that listen to managers club and me, you know, are software engineering managers.
Right. And I believe that AI is gonna have a hugely disruptive effect on software engineering, right. As people write code in the future, future. Mm-hmm. , could you say a little bit in your professional opinion what you see the impact of that? Uh, for people who write code.
Kavita: So I think it’s gonna make us a lot more productive because let’s say you have a code, um, that you to copy [00:20:30] paste from one source to another, you just want that code.
So if you can express that using natural language and get something to like a pseudo code almost, um, that’s gonna save you a lot of time, um, by just reusing that code. But this comes with some problems. Um, some of these tools may have memorized code on the web, so you may be in effect, plagiarizing the code.
So this is not the problem of the software engineers, it’s a problem of the tool itself, [00:21:00] but for software engineers, we can become more productive if it’s even telling you that, Hey, this is, this looks like plagiarized code. Just by using AI behind the scenes, that itself is going to be, um, let’s say you copy pasted code and it detect.
It’s plagiarized code. This is also going to be helpful to you. Um, so that I think there are lots of areas where productivity can be improved. So over time we become more of the designers rather than code writers. So we are basically [00:21:30] putting together blocks of code and designing systems using, uh, AI behind the.
Vidal: That’s great. Yeah. Um, um, Kavita, you’ve been like really generous with your time. This is really interesting topic. Um, where can people reach out to you afterwards if they wanted to learn more about you or, you know, discuss this topic?
Kavita: Sure. Um, if you’re to learn more about me, visit my website, it’s [00:22:00] cavita-ganesan.com, or you can also connect with me on LinkedIn.
So these are the two places where I hang on most and, uh, I’m giving out three free chapters of my, uh, AI book and you’ll find it on my website. And, um, uh, that’s it.
Vidal: Awesome. Thank you so much for coming on. It was great to talk with you. Thank you.